To avoid stifling SME innovation or productivity, the right regulatory balance needs to be struck between Big Tech and UK businesses. Regulation should rightly concentrate on providing assurance that AI systems will be effectively supervised to ensure safety. Equally important is ensuring that UK SMEs who are creating, maintaining or consuming AI systems are not held back or shouldering too much of the burden.
Background
The overall focus of UK AI regulatory aims appears to be evolving to govern those existing developers of commercial foundation models that are powered by generative AI architecture and trained on trillions of data items e.g. Open AI, Amazon Web Services, Google and Anthropic. However, it is remiss to ignore the ‘trickle-down’ impact these regulations, or indeed lack of them, will have on smaller companies employing these foundation models in their own products and/or processes as illustrated below.
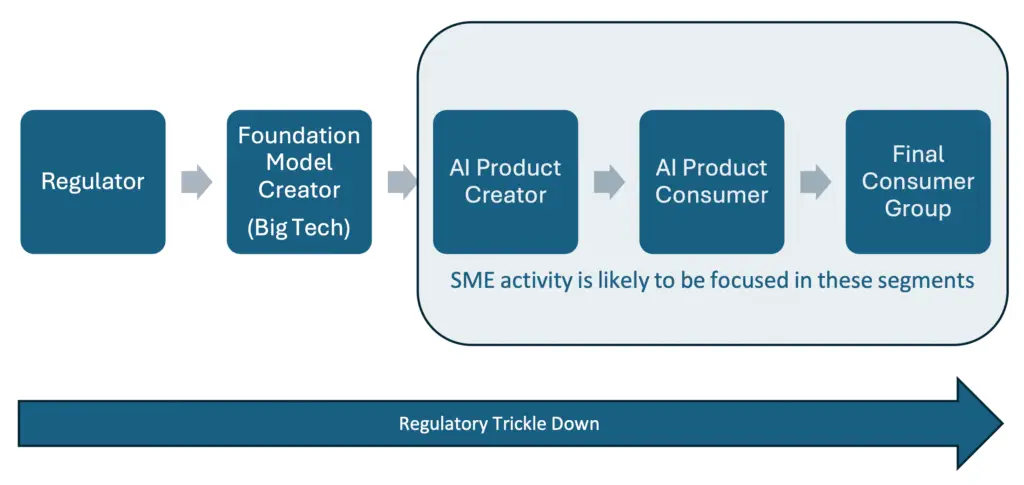
As illustrated by the 3 specific scenarios presented, the practical burden of meeting regulations falls disproportionally on Small and Medium Enterprises (SMEs) in most part due to their small pool of expert resource and smaller amount of readily available capital, especially when compared to the resources of Big Tech. SMEs are the lifeblood of the UK economy with regard to production and the undertaking of innovation in technologically rich areas such as AI. Consequently, there should be specific, informed and practical consideration given to these organisations in any forthcoming regulation of AI systems.
Scenario 1: Disproportionate regulatory burden on SME resource and capital – Data transparency
Transparency and explainability are important targets for any regulation to be effective and practically enforceable. This is commonly referred to as ‘The black box challenge’ as defined succinctly in the House of Lords Members Bill Artificial Intelligence (Regulation) Briefing. Describing and accounting for the data sources, quality, lineage and understanding within an AI system is a concept that has underpinned probabilistic modelling for many decades.
Practically, there is a significant, and often hidden, resource cost to providing such information, especially in the form of a simplified narrative – an approach often favoured in regulation (including the EU AI Act). Data used to train models is frequently complex, from multiple sources and has undergone algorithmic transformation prior to input into model training to improve model performance or to make its format suitable for a specific modelling approach. Consequently, there is likely to be a costly, manual exercise to compress, aggregate and distil the data landscape into a narrative, allowing significant information to be lost and increasing the risk of subjective influence distorting the true properties of the data.
This burden on resource will likely disproportionally and adversely affect SMEs who will not have a large resource pool to call upon, have a more distant relationship with regulatory enforcers and are also more likely to be dealing with data sources less well established than their larger counterparts.
When creating rules and guidance, regulators should ensure that summaries of the AI system training and validation data are requested at the level of model input features and outputs, transformations from source can be supplied in code form (written to common standards) and narrative is used only to provide context to the submission rather than represent the entire data landscape.
Although this approach will mean regulatory enforcement personnel will need to be technically qualified, there will be significant alleviation of resource pressure on SMEs and other companies.
Scenario 2: The impact of a regulatory vacuum on SME’s – Regulatory focus on Big Tech
Recent government commentary on AI regulation has revealed the possibility of regulation focusing on the vendors of the largest foundation generative AI models such as Open AI, Amazon Web Services, Google and Anthropic.
However, a significant danger is the creation of a regulatory vacuum that neglects the role and influence that SMEs, and indeed other companies outside the Big Tech group, can have on the AI landscape and the safety of the public. Take, for example, a small healthcare company that is using the common approach of Retrieval Augmented Generation (RAG) to create a specialised AI agent based on a foundation Large Language Model (LLM) supplied by a Big Tech vendor.
The regulation of the foundation model will ensure the text generated is not subject to significant hallucination or unsafe language and ensures data security. However, the RAG framework created by the SME is not regulated. An SME may lack the resources and capital to properly assess and remediate issues and may not prioritise these checks unless they are regulated. Consequently, errors could result in the agent disclosing incorrect information, ignorant of how well regulated the foundation LLM is, which may be acted on causing harm.
AI regulation must encompass current approaches, such as RAG, and as such address significant risk of unsafe practices in those companies using foundation generative AI models and not just those Big Tech entities that are offering them for commercial use.
Scenario 3: The risks of 3rd party enforcement of AI Regulations onto SMEs – Regulatory focus on
Big Tech
The absence of a hands-on regulator of AI systems combined with a focus on regulating vendors of foundation models may result in Big Tech companies being practically responsible for enforcing regulations on the use of their own models by other companies, including SMEs. This could lead to a conflict of interest if the goals of the SME are in competition with those of the 3rd party Big Tech foundation model vendor.
For example, a small, socially responsible subprime lender wishes to improve the accuracy of their lending assessments by examining narrative information on potential customer behaviour. Collating these data and making them available in concise language at the point of lending assessment can be done using RAG based around a commercially available foundation LLM.
If the vendor of the foundation LLM views the use of their product as high risk in lending to sub-prime customers or at odds with their own values or their larger commercial relationships with established credit reference agencies, a conflict of interest may occur. LLM use by the SME could be restricted, withdrawn or additional regulatory burden placed on the SME by proxy through the LLM vendor. This may adversely impact both the safety and innovation of AI systems.
Key recommendations
- Enforcement agencies should be resourced with experts who are technically qualified and understand the disproportionate burden seemingly simple regulatory requests for transparency may put on SMEs.
- AI regulation must be broadly applied—not just to Big Tech’s foundation models but across those entities using those foundation models in their own applications—in a way that supports innovations across all types of business including SMEs.
- If Big Tech foundation model vendors are to play a part in regulating entities who use their models, then there should be a robust and fair framework to avoid conflicts of interest.
Contributors
Lord Iain McNicol, Lord Kulveer Ranger, Gordon Baggott (AI Director, 4 Most Europe Ltd),
Richard Davis (CEO, Inference Group Ltd), Mark Somers (CPO, Fifty One Degrees AI Ltd),
Colin Carmichael (Partner, 4 Most Europe Ltd), Adam Leon Smith (Chair, AIQI Consortium)